Food donation forecasting is a critical aspect of food bank operations, helping to ensure that donations align with demand and reduce waste. Predictive analytics offers a powerful tool for improving the accuracy of these forecasts, optimizing resource allocation, and enhancing the overall efficiency of food distribution networks. This article explores how predictive analytics is transforming food donation forecasting and highlights its benefits, challenges, and implementation strategies.
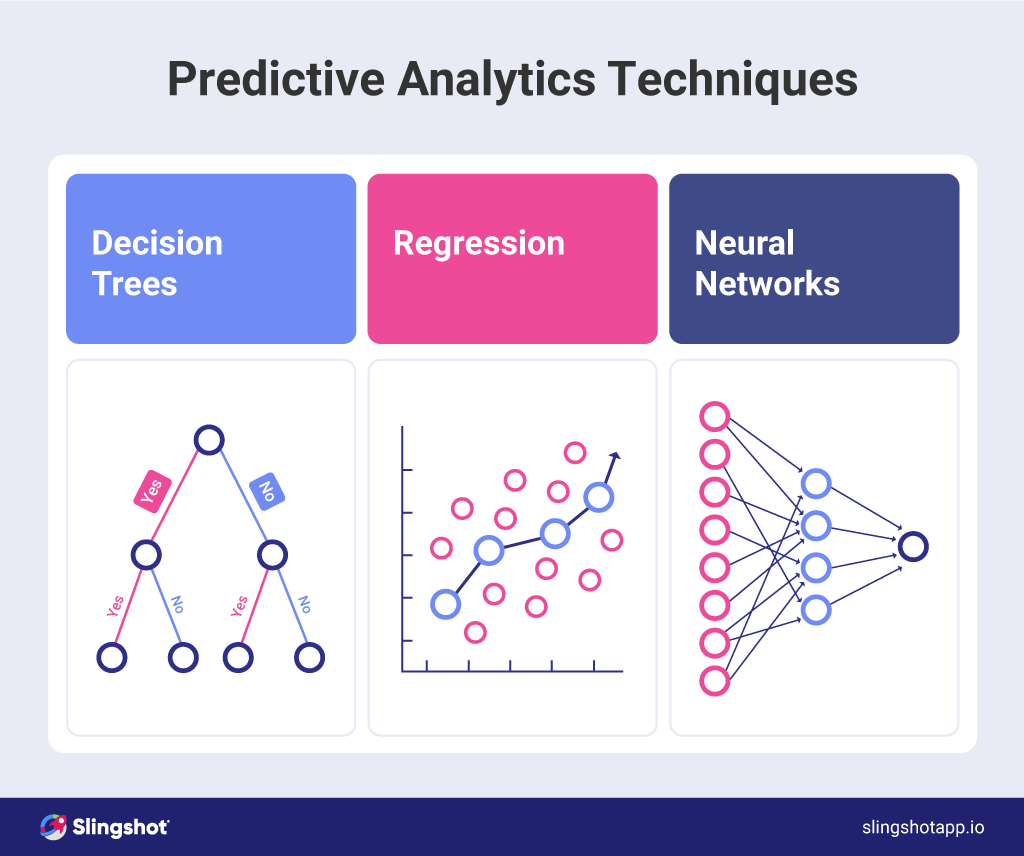
Understanding Predictive Analytics
Predictive analytics involves using historical data, statistical algorithms, and machine learning techniques to predict future outcomes. In the context of food donation forecasting, predictive analytics helps food banks anticipate the quantity and types of food donations they will receive, as well as the demand for these donations. By leveraging data from past donation patterns, economic indicators, and community needs assessments, food banks can make informed decisions to optimize their operations.
Benefits of Predictive Analytics in Food Donation Forecasting
1. Improved Accuracy in Forecasting
One of the primary benefits of predictive analytics is the ability to enhance forecasting accuracy. Traditional forecasting methods often rely on manual estimates or simple trend analysis, which can lead to inaccuracies and inefficiencies. Predictive models, on the other hand, use complex algorithms to analyze multiple variables simultaneously, resulting in more precise predictions.
For instance, by analyzing data on seasonal donation trends, economic conditions, and community events, predictive models can forecast periods of high or low donations. This enables food banks to prepare accordingly, reducing the risk of overstocking or shortages.
2. Optimization of Resource Allocation
Accurate forecasts allow food banks to allocate resources more effectively. Knowing when and what type of food donations to expect helps food banks plan their logistics, including storage, transportation, and distribution. For example, if a predictive model forecasts a surplus of perishable items, the food bank can prioritize their distribution to ensure they are used before expiration.
Moreover, predictive analytics can assist in volunteer management by forecasting the manpower needed for sorting, packing, and distributing food based on expected donation volumes.
3. Enhanced Donor Engagement and Planning
Predictive analytics can also play a crucial role in donor engagement. By understanding donation patterns, food banks can identify potential high-impact donors and tailor their outreach efforts. Predictive models can suggest the best times to reach out to specific donors or launch fundraising campaigns, maximizing the effectiveness of these efforts.
Additionally, food banks can use predictive insights to provide feedback to donors on how their contributions are likely to impact the community, encouraging more consistent and targeted donations.
4. Reduced Food Waste
Food waste is a significant issue for food banks, particularly when dealing with perishable donations. Predictive analytics helps mitigate this problem by forecasting donation volumes and types more accurately, enabling better inventory management. For example, if the model predicts a large influx of fresh produce, the food bank can coordinate with partner organizations to distribute these items quickly, reducing spoilage and waste.
Key Components of Predictive Analytics in Food Donation Forecasting
Implementing predictive analytics in food donation forecasting involves several key components:
1. Data Collection and Management
The foundation of any predictive analytics model is high-quality data. Food banks need to collect and manage data on various factors, including:
- Historical Donation Data: Information on past donations, including quantity, type, and source.
- Demand Data: Records of food distribution and community needs assessments.
- External Factors: Economic indicators, weather conditions, and local events that may influence donation patterns.
Effective data management systems are essential for storing and organizing this information in a way that is accessible for analysis.
2. Model Development and Training
Once the data is collected, the next step is developing and training predictive models. This process involves selecting appropriate algorithms and using historical data to train the model. Commonly used techniques in predictive analytics include:
- Regression Analysis: Used to identify relationships between variables and predict donation volumes based on historical patterns.
- Time Series Analysis: Analyzes data points over time to forecast future trends, particularly useful for identifying seasonal patterns in donations.
- Machine Learning Models: Advanced models, such as decision trees and neural networks, can analyze complex data sets with numerous variables to generate more accurate predictions.
Model development also involves continuous training and validation to improve accuracy over time.
3. Implementation and Integration
Implementing predictive analytics in a food bankβs operations requires integrating the models into existing systems and workflows. This includes:
- Inventory Management Systems: Integrating predictive models with inventory management systems allows for real-time updates and adjustments based on forecasted donations.
- Logistics Planning Tools: Using predictive insights to optimize transportation and distribution routes, ensuring efficient use of resources.
Successful integration requires collaboration between data analysts, IT professionals, and food bank staff to ensure the system meets operational needs.
Challenges in Implementing Predictive Analytics
While predictive analytics offers numerous benefits, there are several challenges associated with its implementation in food donation forecasting:
1. Data Quality and Availability
Accurate predictive models depend on high-quality data. Food banks often struggle with incomplete or inconsistent data, which can compromise the reliability of forecasts. Addressing this challenge requires robust data collection and management practices, including standardized data entry protocols and regular data audits.
2. Resource Constraints
Implementing predictive analytics can be resource-intensive, requiring investments in technology, training, and personnel. Many food banks operate with limited budgets and may lack the financial resources or technical expertise needed to develop and maintain predictive models. Partnerships with academic institutions, tech companies, or data analytics firms can provide the necessary support and expertise.
3. Resistance to Change
Integrating predictive analytics into food bank operations may face resistance from staff accustomed to traditional methods. Overcoming this challenge requires clear communication about the benefits of predictive analytics and providing training to ensure staff are comfortable using new tools and systems.
Case Studies and Success Stories
Several food banks have successfully implemented predictive analytics to enhance their operations. For example, Feeding America has developed a network-wide predictive analytics platform that helps member food banks forecast demand and manage inventory more effectively. This platform uses data on economic trends, population demographics, and historical distribution patterns to provide actionable insights that improve food distribution efficiency.
Another success story comes from the North Texas Food Bank, which uses predictive analytics to optimize its supply chain and reduce food waste. By analyzing data on donor behavior and community needs, the food bank has been able to increase the accuracy of its forecasts, resulting in better resource allocation and reduced operational costs.
Future Directions for Predictive Analytics in Food Banks
The use of predictive analytics in food donation forecasting is still evolving, with several emerging trends likely to shape its future:
1. Integration with Real-Time Data
The integration of real-time data, such as weather forecasts or social media trends, can further enhance the accuracy of predictive models. For example, anticipating spikes in demand due to natural disasters or economic changes allows food banks to respond more proactively.
2. Use of Artificial Intelligence
Advanced AI techniques, such as deep learning, have the potential to improve the predictive accuracy of food donation models. AI can analyze larger and more complex data sets, providing deeper insights into donation and demand patterns.
3. Expansion to Global Food Bank Networks
While predictive analytics has primarily been used in the United States, there is potential for its application in food banks worldwide. Expanding these tools to global networks can help address food insecurity on a broader scale, ensuring more efficient food distribution in regions facing severe hunger and poverty.
Conclusion
Predictive analytics offers a powerful solution for managing food donation forecasting, helping food banks optimize their operations and better serve their communities. By leveraging data-driven insights, food banks can anticipate donation trends, allocate resources more efficiently, and reduce food waste. As technology advances and more food banks adopt predictive analytics, the potential for improving food security and reducing hunger will continue to grow.
For more information on the use of predictive analytics in food banking, visit Feeding America and explore their resources on food donation and distribution management.
Leave a Reply